Many senior executives are fascinated by and anxious about Artificial Intelligence (AI). While the potential for AI to transform business operations is clear, it also presents challenges.
In the rush to embrace artificial intelligence, many organizations are putting the cart before the horse. While AI's transformative potential is undeniable, most companies aren't ready to capitalize on it. The good news is that the steps required to become AI-ready align perfectly with building a stronger, more data-driven organization overall.
The AI Readiness Paradox
The greatest danger in today's AI landscape isn't skepticism—it's blind enthusiasm. When everyone agrees that something will be transformative, it's often a clear sign that we're missing critical nuances. The real challenge isn't the technology itself but rather our organizational readiness to leverage it effectively.
Many organizations rush to adopt AI, driven by fear of missing out or being left behind by competitors. In this rush, executives forget that technology is not a magic button. Like any tool, AI requires strategy, proper data structures, and process maturity to deliver value. If AI implementation is misaligned with business processes, it can create more confusion than clarity, potentially derailing existing operations.
This creates a complex paradox: While it would be foolish to think AI won't be a game-changer, rushing to implement it without proper preparation could be equally detrimental. The key is finding the right balance between progress and preparation.
The Five Levels of AI Maturity
To understand where your organization stands, consider these five levels of AI maturity:
1. Piloting & Experimentation: Testing and learning with isolated use cases
2. Isolated Usage: Implementing AI in silos across different departments
3. Partial Synchronization: Coordinated AI implementation across parts of the organization
4. Full Synchronization: Connected AI implementation across the entire organization
5. Integration: AI becomes part of the organizational operating system
Here's the reality check: 98%+ of organizations are still at Level 1, and that's okay. The critical mistake is jumping directly to Level 3 or 5 without laying the proper groundwork.
Are you rushing to implement AI without the proper foundation? You're not alone.
Here's the good news: the steps to become AI-ready align perfectly align with building a stronger, more data-driven organization.
We're putting on an exclusive executive webinar designed to cut through the AI hype and deliver actionable insights on building true AI readiness in your organization. Join us on December 5th at 2pm ET so you can be prepared to move from AI experimentation to implementation with clarity and confidence.
The Prime Directive of AI Implementation
Before diving into technical requirements, remember this fundamental principle: AI should never drive strategy – strategy should drive AI. Similarly:
- Business processes should drive AI, not the other way around
- AI should enhance human thinking, not replace it
- AI should be a method of execution, not the job itself
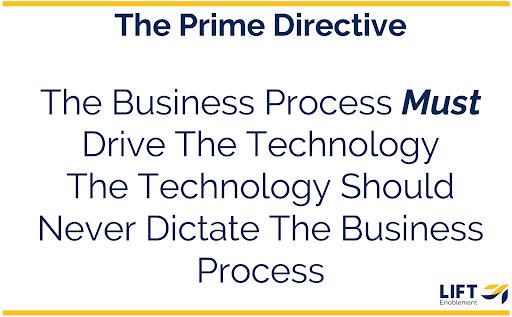
Critical Components of AI Readiness
1. Data Architecture and Quality
The foundation of AI readiness isn't in the algorithms – it's in your data. AI is only as effective as the data it processes. Poor data quality or disorganized structures limit AI's potential, leading to inaccurate insights. Many businesses struggle with “mile-wide but inch-deep” datasets that lack the depth for meaningful analysis.
You must focus on building robust data architectures. This includes identifying critical data points, cleaning existing datasets, and establishing data governance practices. Investing in data management tools and processes now will set the foundation for AI to deliver actionable insights in the future.
To be AI-ready, you need:
- A hierarchical ERD (Entity Relationship Diagram) that clearly defines data relationships
- A clear understanding of data layers, roles, and lanes
- Both quantitative and qualitative data capture
- Real-time data logging (not just retrospective entry)
- Proper data validation and structure
Remember: More than half of critical business interactions happen offline, and your data architecture needs to account for this reality.
2. Business Process Maturity
Before implementing AI, companies must refine their business processes. Automating broken or inefficient processes only amplifies existing problems. “You can’t automate what you haven’t done manually,” said Jess Cardenas, emphasizing the need for companies to understand their workflows fully before automating them.
For organizations in the lower stages of process maturity, AI can help automate repetitive, low-value tasks. However, human oversight is essential to ensure AI solutions align with the intended outcomes and evolve as the business grows.
Before implementing AI, assess your business process maturity. Key questions include:
- Are your processes well-defined and documented?
- Do you have established systems, playbooks, and methodologies?
- Can you identify clear gaps where AI could add value?
- Have you manually executed the processes you want to automate?
3. Technical Infrastructure
AI is not a substitute for strategy; instead, it should align with and support your existing business strategy. Senior leaders should avoid the temptation to treat AI as a shortcut. Successful AI integration requires companies to clarify their strategic objectives first and determine where AI fits them. AI becomes a costly experiment rather than a performance enhancer without this alignment.
Your technology stack needs to support AI implementation:
- Ensure systems are fully connected and integrated
- Evaluate vendor AI policies and capabilities
- Consider data security implications
- Assess training data requirements and ownership
4. People and Skills
AI implementation is as much about people as it is about technology. Successful adoption requires leadership, training, and change management to help employees understand and embrace new ways of working. Companies need to focus on developing the right skills within their workforce to leverage AI effectively.
Learning and development programs should teach employees not only how to use AI tools but also how to interpret their outputs. Critical organizations might become passive participants, relying too heavily on AI-generated insights without questioning their validity.
The human element is crucial. Focus on:
- Building a data-driven culture
- Developing AI literacy across the organization
- Determining whether you'll be an AI creator, consumer, or both
- Investing in learning and development programs
- Creating clear AI policies and ethical guidelines
Moving Forward: Practical Steps for Manufacturing Leaders
1. Conduct an AI Readiness Assessment:
Evaluate your organization's position on the AI maturity scale and identify gaps in data, processes, and skills.
2. Develop a Roadmap for AI Adoption:
Create a phased plan that aligns with your business strategy. Start with small experiments and gradually scale AI solutions.
3. Focus on Data Management:
Invest in tools and practices to improve data quality and ensure your datasets are structured for AI analysis.
4. Build Internal Expertise:
Provide training and resources to help employees become comfortable with AI tools and develop critical thinking skills around AI-generated insights.
5. Adopt a Growth Mindset:
Encourage experimentation and learning. Treat AI as an evolving tool that requires ongoing refinement and adaptation.
6. Start Small:
Begin with high-effort, low-value activities where AI can demonstrate clear ROI.
7. Focus on Enhancement:
Use AI to make your people better, not replace them.
8. Build Confidence:
Help your team see AI as an enabler rather than a threat.
9. Maintain Control:
Ensure you're driving the AI, not letting it drive you.
10. Stay Strategic:
Connect all AI initiatives to clear business objectives.
The message is clear: While AI will be transformative, the winners will not be those who implement it first but those who implement it correctly. The good news is that the steps required for AI readiness—better data architecture, mature business processes, strong technical infrastructure, and skilled people—are the same for building a stronger organization overall.
Keep FOMO (Fear of Missing Out) from driving your AI strategy. Instead, focus on building a solid foundation. If you're at the experimentation stage, embrace it. Use this time to prepare your organization for the AI-driven future while continuing to deliver value through traditional means.
Remember: AI readiness isn't a destination—it's a journey. The organizations that will succeed are those that approach this journey with clear eyes, strong fundamentals, and a commitment to doing things right rather than just fast.
The question isn't whether AI will transform manufacturing—it's whether your organization will be ready for it when it does.